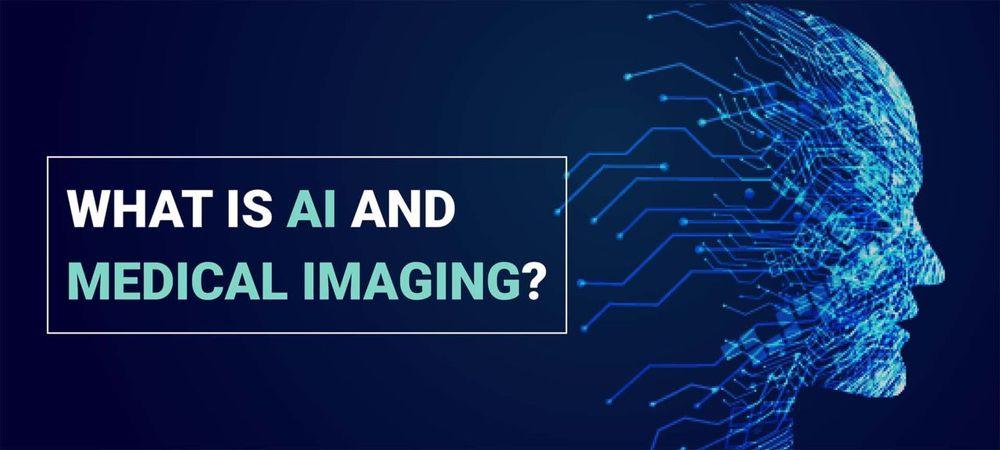
Artificial Intelligence or simply AI- the ability of a computer to work by its own-trained intelligence was able to make a huge impact on the community by now. AI plays a huge role in the field of business management, transport, and automobile industries. There is no doubt that AI makes a devastating impact transmitting processes from human interaction to computer-based interactions.
Images on medical testing are huge sources of information on the field of health care while it was considered a difficult way to process. Clinicians and radiologists today specially process a large number of images to diagnose a disease. However, this process needs a skilled human resource. With the growth of the population, it was a necessity to automate this diagnosis by a pre-trained machine such that more accurate and validated results were given. This helps the patients to have immediate treatments and the ultimate goal is to have a better service for the public.
Unlike in other fields, there was a little doubt and a lot has been said that the use of AI in the health sector, especially in disease diagnosis has not produced a successful implementation. This was especially true in the field of medical imaging. This article may help to have an understanding of how the analysis was performed and how it validates the results produced to make a discussion that AI CAN make a viable solution to the stated attitude.
Global influence in medical imaging:
Globally the medical imaging market size by 2019 was estimated as USD 2.14 billion. By the year 2021, it has reached a value of USD 2.60 billion.
Who benefits from image analysis with AI?
The following group of people will get a benefit from the image analysis automation process.
- Radiologists
- Non- radiologists
- Patients
Apart from that, the following benefits can be gained by using AI for medical image analysis.
- Improve health outcomes
- Improve health quality
- Rapid and accurate diagnosis
- Saving time
- Saving money
- Remedy for the lack of human resources
Sources of images:
The most recent equipment used for scanning includes Magnetic Resonance Imaging (MRI), Ultrasound (US) scanning, Angiography and Computed Tomography (CT), Positron Emission Tomography (PET). These are also important for diagnosis as well as follow who is undergoing treatment. Most of these technologies are based on X-ray and Ultrasound wave reflections.
The following images reflect the idea of image processing using the above scanners.
The US scanners and X-ray images project X-ray beams into the human body. Some of the parts of this radiation are absorbed and some are reflected. Based on this reflected beam the images are generated.
However, the MRI, CT, PET scanners do not ionize the medium when taking images.
Areas of application:
- Dental -- Detection of teeth -- Detection of filled teeth -- Detection of crowns -- Detection of prosthesis
- Neurology -- Identify psychiatric disorders -- Identification of strokes
- Urology -- Predicting urological diseases
- Oncology
- Gynecology
Why is image analysis important?
Generally, the MRI, CT, US-scan reports are analyzed and processed by radiology experts and their opinion is passed into a physician according to the field of expertise for further treatments.
However, with the growth of the population, a huge number of images related to these scanning processes are produced. Nevertheless, training and producing an expert radiologist consumes considerable time. Therefore AI produced an impressive solution for this health problem.
“How if the predictions are produced with the medical imaging process?”
How image analysis is done?
Let us discuss how the analysis is done. First, we need a sample image dataset with a considerable number of records to model, test, and validate the model. Therefore, the dataset looks like this;
Then
the dataset is needed to be preprocessed. The horizontal, vertical flipping, rotations, zooming, setting of brightness and contrast, and the dataset are transformed, as we wanted. Then the Convolution Neural Network architecture was created. The following images describe how CNN works.
Evaluate model accuracy:
It is necessary to measure how much the model predicts a given image accurately. Therefore to measure this accuracy level of a developed classification machine-learning model a confusion matrix is used. In a confusion matrix, the testing data are either classified according to the state of how they are classified, a correct classification or incorrectly classified. The confusion matrix can be visualized as below.
The model accuracy is evaluated using the confusion matrix and the following formula.
For a better classification model, it is assumed that it takes at agoradesign.it least an accuracy of 80%.
Used Case Scenarios:
1. Classification model for the detection of Covid-19, Pneumonia, Lung cancer, and chest diseases.
In this study, several pre-trained models are used to classify a given chest X-ray image into 4 classes; Covid-19 Pneumonia, Chest disease, and Lung cancer. The following two basic models are used in the feature engineering process.
- VGG19 + CNN model
- ResNet152V2 model.
The dimensions of each layer in the network are mentioned by the authors.
The confusion matrix was used to identify the accuracy of each model.
This process needs a bit high system requirements, storage capacity, and high processing power.
2. Dental image scanning
Projects that have been completed using image analysis:
Leading organizations expert in clinical image analysis:
- CALYX
- World care clinical
- GE Healthcare
- AQUILAB
- MIM software INC.
Rhino Partners
We are South East Asia's best software development and data science company providing customised solutions for fintech clients worldwide.